Image upscaling technology has evolved dramatically, with REAL ESRGAN emerging as a ground-breaking advancement in this field. Building upon the foundational concepts of ESRGAN, REAL ESRGAN focuses on transforming low-resolution images into stunning, photorealistic visuals. This algorithm addresses the unique challenges of noise, distortion, and imperfections by utilizing sophisticated deep-learning techniques and extensive training datasets of real-world photographs.
This article delves into the intricacies of REAL ESRGAN, highlighting its key improvements and practical applications for enhancing image quality.
In this article
Part 1. What is REAL ESRGAN?
REAL ESRGAN refers to an advanced image upscaling algorithm built upon the foundation of Enhanced Super Resolution Generative Adversarial Networks (ESRGAN). Originally developed to enhance the resolution and quality of images while preserving or even enhancing details, REAL ESRGAN brings a focus on producing more photorealistic images. The algorithm utilizes deep learning techniques, specifically convolutional neural networks (CNNs), to reconstruct an image at a higher resolution, making it appear sharper and more detailed than its lower-resolution predecessor.
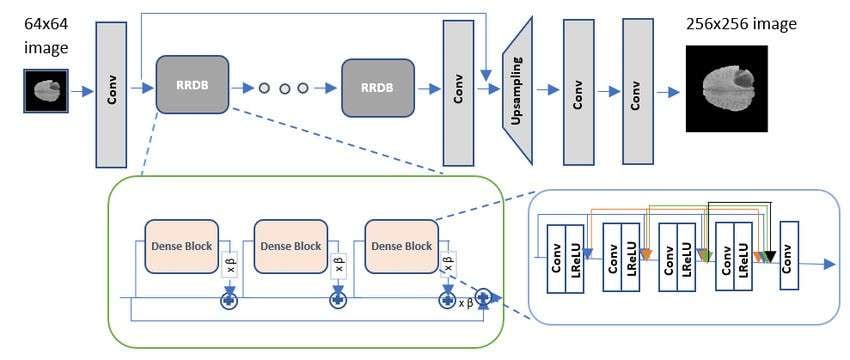
Unlike traditional upscaling methods that often introduce blurriness or artifacts, REALESRGAN aims to improve the quality by learning from a diverse dataset comprising artistic and photographic styles. The model's architecture involves two main components: a generator that creates high-resolution images from low-resolution inputs and a discriminator that evaluates the authenticity of the generated images. This back-and-forth training approach enables REAL ESRGAN to refine its techniques continually, producing results that are convincing even to seasoned observers.
Part 2. Difference between ESRGAN and Real-ESRGAN
The primary distinction between ESRGAN and REAL ESRGAN lies in the evolution of the architecture and approach to generating high-resolution images. ESRGAN, developed initially as an enhancement over the existing super-resolution networks, focuses on improving visual quality by employing a generative adversarial network (GAN)-based mechanism. Its primary function is to upscale images while attempting to retain finer details and textures, driven by a loss function that highlights perceptual quality.
REAL ESRGAN, on the other hand, takes these principles further by enhancing the algorithm's capabilities to deal with real-world images more effectively. It leverages more sophisticated training techniques, including enhanced datasets that include a more diverse range of images, including those affected by real-world factors such as noise and blurriness. This adaptation means that REALESRGAN can produce results that are not only of higher resolution but also more photorealistic, allowing it to tackle complexities and variations found in actual photographs rather than just stylized or synthetic inputs.
Part 3. How Real-ESRGAN Went from Synthetic to Real-world Images?
The transition of Real-ESRGAN from handling synthetic images to effectively processing real-world images marks a significant milestone in the field of image super-resolution. Initially, ESRGAN was primarily trained on synthetic images, designed to demonstrate its capability to enhance and create high-resolution outputs. However, this limited focus made it less adept at addressing the unique challenges posed by real-world photographs, such as imperfections, varying image qualities, and inherent noise.
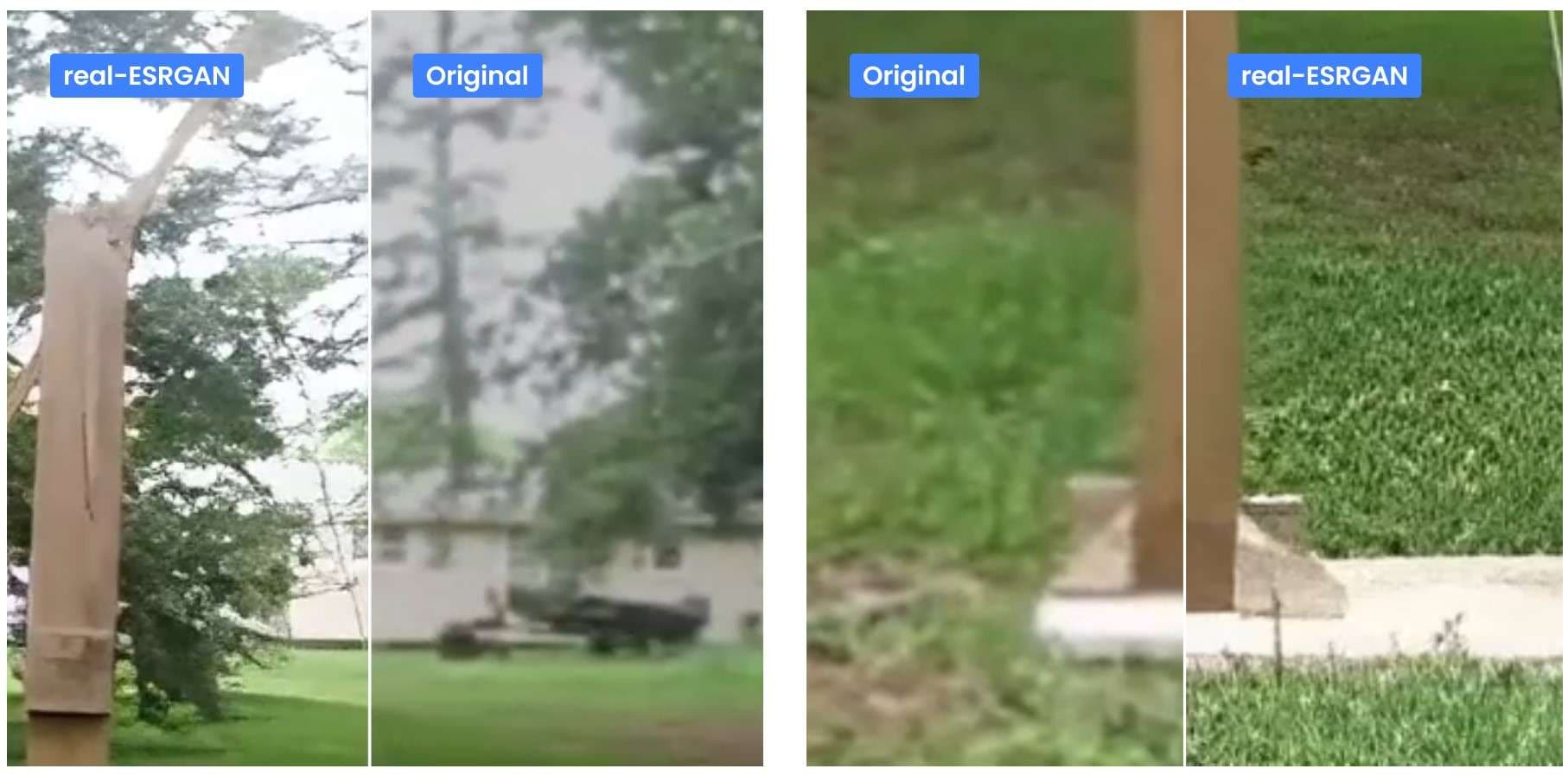
To bridge this gap, researchers developed Real-ESRGAN by incorporating a more robust training dataset that included millions of images sourced from real-world environments. By using a diverse array of photographs ranging from landscapes to urban scenes, the model learned to recognize and adapt to the complexities of real-world scenarios. This involved training on images with varying levels of degradation, such as compression artifacts and low lighting conditions. Additionally, the algorithm integrated advanced techniques, including conditional adversarial training, to better evaluate and reconstruct complex textures and details found in actual photographs.
Part 4. Key Improvements in Real-ESRGAN
Real-ESRGAN introduces several key improvements over its predecessor, ESRGAN, significantly enhancing its ability to create high-fidelity images from lower-resolution inputs. Firstly, the architecture has been refined to better manage the complexities associated with real-world imagery. This includes sophisticated disentanglement of content and style, enabling the model to separate features more effectively, which leads to more accurate detail reconstruction in the output images.
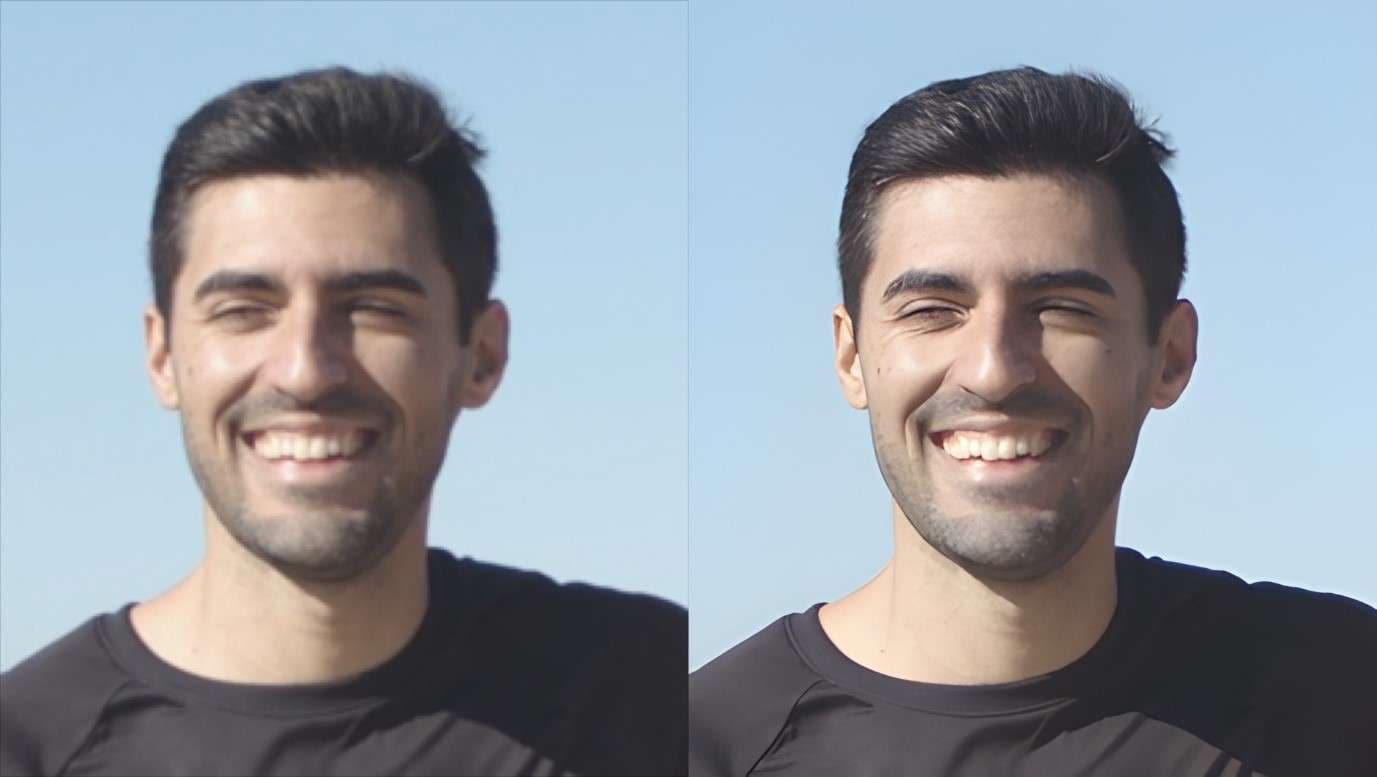
Another critical enhancement is the incorporation of advanced training strategies using better dataset diversity. Real ESRGAN online is trained on a wider range of images, including various resolutions, levels of distortion, and types of degradation. This extensive dataset allows the model to learn how to handle and correct the imperfections found in real-world images, leading to enhanced quality and authenticity in the generated output. The model also integrates improved normalization techniques, which help stabilize training and enable the model to generalize better across different types of images.
Bonus Tip: Use Repairit for Enhancing Images
Repairit Photo Enhancer is a highly dependable tool for enhancing images through its advanced algorithms and user-friendly interface. It employs AI-powered technology to seamlessly repair blurry, noisy, or damaged photos, restoring them to their former glory. The intuitive design guides users effortlessly through the repair process, ensuring optimal results even for beginners.
Repairit's commitment to continuous updates ensures its compatibility with various image formats, significantly streamlining the image enhancement workflow. Repairit empowers users to revitalize their cherished memories and elevate their visual content to new heights by leveraging its exceptional capabilities.
Step 1: Begin by downloading and installing Repairit. Navigate to the "AI Photo Enhancer" tab within the program's interface to initiate the process.
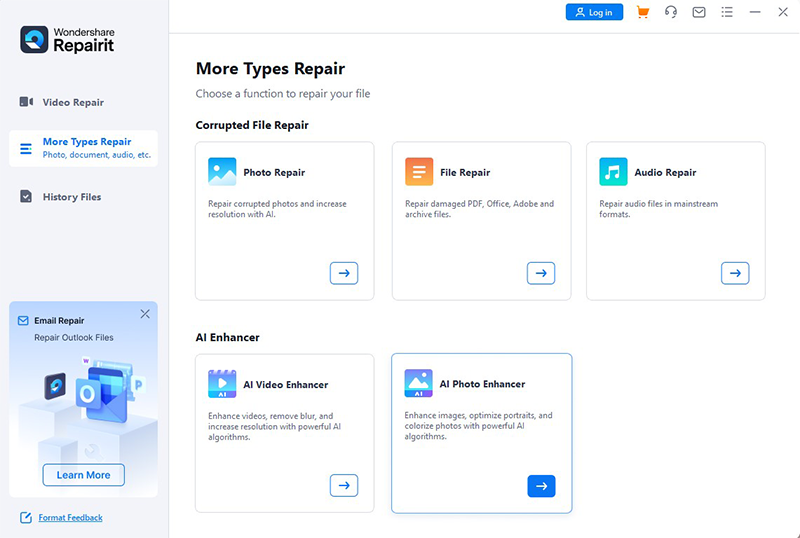
Step 2: Select the images you wish to enhance and upload them into the program. Repairit's AI technology will analyze and automatically enhance your photos.
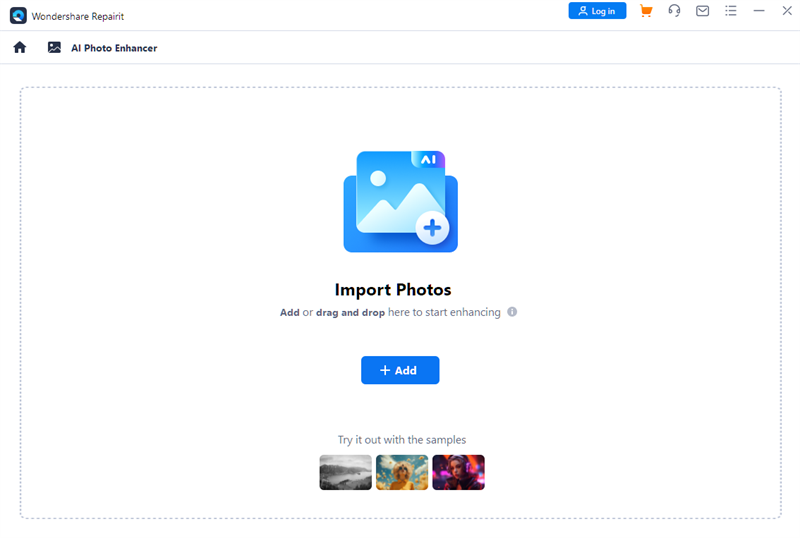
Step 3: Preview the transformed images to ensure satisfaction. Subsequently, select the desired output and click the "Save" button to preserve the enhanced photo.
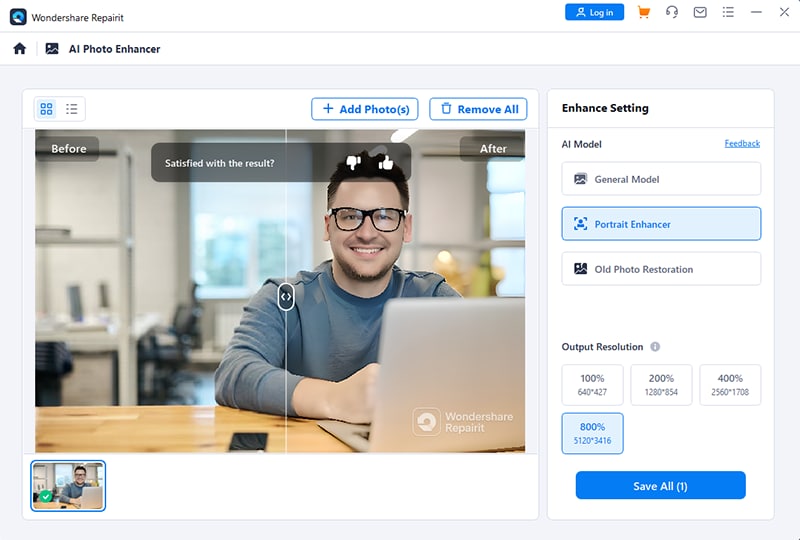
Enhance Your Video To Next Level
Security Verified. Over 7,302,189 people have downloaded it.
Conclusion
REAL ESRGAN represents a significant advancement in image upscaling technology, transcending its predecessor by effectively addressing real-world complexities. Its enhanced capabilities and training methodologies allow for producing high-fidelity, photorealistic images. Repairit is a trustworthy tool for enhancing images fast and effectively.
FAQ
-
Is Real-Esrgan Good?
Real-ESRGAN is a highly regarded image upscaling tool that utilizes deep learning to enhance image resolution while maintaining quality. It is particularly effective at restoring details in both natural and synthetic images. -
How to Install Real Esrgan on Windows?
To install Real-ESRGAN on Windows, you can use pre-built executable files or install it via a Python environment with dependencies like PyTorch and OpenCV. Detailed instructions are often found in the official GitHub repository, guiding you through setup and usage.